Artificial intelligence is more than a buzzword when it comes to marketing. While there's still plenty of unfulfilled AI promise (no robotic creative directors in sight), it's also true that sophisticated marketers are using AI right now — and delivering results.
Sure, we all know about AI-powered tech, like chatbots, voice recognition and automated ad bidding. But there are other, more direct applications of AI in marketing quietly driving the decisions of some brands.
Right now, marketers are using AI to tackle challenges like:
1. High-value customer segmentation: What characteristics define my most valuable customers, and how can I find more of them?
2. Churn risks: Which customers are most likely to churn, and how can I retain them?
3. Product propensity: Which consumers are most likely to accept my latest offer?
Answering these questions requires examining why a consumer behaves a certain way. Whether we seek to identify the common characteristics of high spenders, churn risks or high-propensity audiences, we must paint a picture of the consumer and understand the attributes (known as "features" in data science) that influence the way they behave. Marketers can use AI to build these portraits. Specifically, AI offers a framework to build predictive models that are powered by the attributes that predispose consumers to behave a certain way.
AI For Targeted Marketing
Here is a walkthrough of the predictive modeling process as it relates to marketing to shine a light on how consumer attributes can be used to predict future behavior.
Data scientists apply AI techniques (machine learning and predictive modeling) on consumer data to figure out which consumer characteristics are most likely to be associated with a specific behavior. The resulting characteristics are considered to be "predictive features."
Predictive features can be created from demographic characteristics (age, gender, profession and marital status), or behavioral characteristics (international traveler, marathon runner or high-end shopper). If you choose them carefully, the right set of features can identify whether (or not) someone is likely to take certain actions.
The "action" depends on marketing goals, and it could be either a desired outcome or one that needs to be prevented: booking a trip, signing up for a particular kind of service, account cancellation and so on.
With a carefully selected set of predictive features in hand, data scientists can use AI techniques to analyze past behavior and find patterns that are predictive of the outcomes they want to drive.
Case In Point: AI's Predictive Characteristics In the Field
To understand in more detail how this actually works, let's look at a real-world example of a food delivery company (a former client of my company, an AI- and data-based consumer intelligence solution). With the goal of growing its revenue, this company sought to better understand its highest-value customers, increase the percentage of these valuable customers within its customer base and develop acquisition and retention strategies for them.
To achieve this, the company employed a predictive modeling technique known as recency, frequency and monetary (RFM) modeling. RFM modeling helped define the predictive features of a high-value customer in a manner that created actionable insights for marketing campaigns.
Step 1: The Starting Point
Like most businesses, the food delivery company maintained robust data about its customers and their order history. In data science, this internal data is known as first-party data. Marketers need to start by understanding the first-party data available to them in their organizations and taking an inventory of what they have access to. Depending on the organization, access to data is highly variable. There will be some data that is readily available and unfortunately, there will be data that you will never get your hands on.
First-party data is incredibly valuable, but it does not typically provide the kinds of insights needed for efforts like audience segmentation or programmatic ad buying. For the delivery company, it was relatively easy to define its best customers using first-party data, but these "best customer" characteristics couldn't be applied to the general consumer population. You cannot create a market segment of middle-aged women who like to order Italian food after 7:00 p.m. (although I could be over-personalizing this).
Step 2: Data Enrichment And Feature Selection
After inventorying the data that is available to you, determine where you have gaps. What sort of information would be useful to better understanding customers and prospects? What data elements will build that elusive 360-degree profile?
In our example, by enriching its first-party data with third-party consumer data (external data acquired through a data marketplace), the food delivery company was able to create a fuller picture of the customer and generate a set of predictive features that defined its best potential customers: young (25-34 years old) couples with kids in which both partners worked and had lengthy commutes.
Step 3: Taking Action On Insights
Once you have enriched your data, you are ready to act. You can use this data as part of your predictive modeling, advanced segmentation and personalization to increase the effectiveness of your efforts.
Predictive features in hand, the food delivery company worked with their marketing partners to identify and target audiences of dual-income professionals who had kids and long commutes, which made it possible to send campaigns directly to the people who were most likely to become high-value customers.
The AI Payoff
The application of AI in marketing is a nuanced process that requires the right volume and type of consumer data. It can be less time-consuming and substantially more effective than traditional methods. Gathering the depth, breadth and scale of data for AI is just one of the challenges (but possibly the biggest) that marketers may encounter. Another common challenge is with internal understanding and skill sets. Does your organization really understand the benefits of AI, and do you have the right people to successfully to implement it? Finding a trusted partner for the near term is one way to overcome these challenges if you lack internal resources.
While AI can seem intimidating, hopefully this explanation demystifies the overlap between marketing, data science and machine learning. Marketers who team up with data scientists to use AI to determine predictive attributes and create predictive models can yield impressive campaign results.
This article originally appeared on Forbes.com.
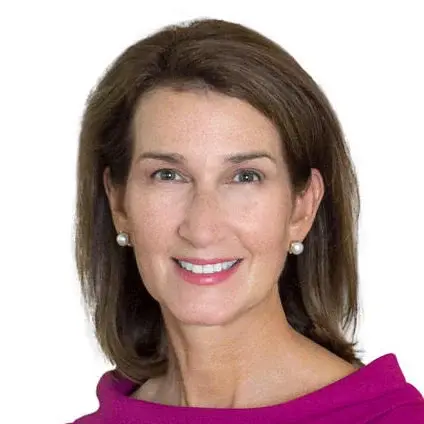