Predictive models help predict future behaviors, and are powerful tools for supporting business decisions and improving operations.
But predictive models are only as accurate and reliable as the data that powers them.
In this episode of Data Point of View by Anindya Datta, CEO & Founder of Mobilewalla discusses the challenges of model performance degradation in production, how to tackle data accuracy issues, and the role of data stability in building resilient models.
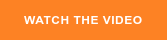
Key Insights
Data drift is the most common reason for problems of resiliency in models.
- While there are many possible reasons why models might perform poorly in production, data drift is the main one. One of the main reasons that models in production behave differently than when they're trained and tested is changes in the properties of the data that anchors these models. The original data used to create the features on which the model was trained differs from those that power the model in production. Usually this happens when some time has elapsed since the model was deployed and, in that time, the nature and properties of the data powering the features that are anchoring the model also change.
We need to build resilient machine learning models from scratch.
- Even though there are effective ways of measuring data drift, these don't help build features and models that are resilient from scratch. Without resiliency, operationalizing machine learning models would remain a major challenge. Modelers will continue to build hundreds of models, which will underperform in production and require frequent correction. And the continual need to re-engineer these models will raise organizational doubts and questions over the operational utility of machine learning and predictive modeling.
Data stability is a powerful tool for building and maintaining resilient models.
- To build resilient models, you need to anchor them with data that doesn't often drift. This is called data stability. To build resilient models, we need to find data, or we need to be able to identify the drift properties of data so that we can preferentially build a model with data that drifts less. At Mobilewalla we call this stability. Stable data is data that drifts less, and while drift is a point measure, stability is a longitudinal metric. Stability is the property-specific data attribute that doesn't drift a lot over time."
