The market for machine learning (ML)and artificial intelligence (AI) is booming and is affecting nearly every industry. In two years, the total global revenue for AI software, hardware, and services is projected to reach $554 billion. In 2021, that figure was an estimated $327 billion. The AI-driven future is no longer on the horizon. It’s here.
In this world, data scientists and line-of-business leaders cannot rely on their company’s first-party data and build predictive and resilient models. And while enriched third-party data that’s deeper and broader than what a company has collected on its own is a potential boon to ML and AI, this raw data alone does not power the insights that give predictive models value.
That’s where feature engineering comes in.
What is Feature Engineering, and What Role Does It Play In Predictive Modeling?
A predictive model is a combination of attributes or variables (also known as features) and algorithms (machine learning code) that predict the likelihood of an outcome. Feature engineering, the transformation of data into these attributes or features, is a crucial part of predictive modeling success.
Feature engineering is the process of transforming raw data into features and identifying the most predictive attributes to use in modeling. Feature engineering supports the creation of predictive models that produce actionable insights in business use cases, such as propensity to churn or acceptance rates for a product offer.

How is Feature Engineering Performed?
Feature engineering is typically performed manually by data scientists. Guided by intuition, it’s an overtly human step in a machine-centric field. Since a given data set may have a countless number of possible features, it’s not reasonable for even the most sophisticated algorithms to compute and evaluate them all. Human intervention guides the process towards what are hopefully the most meaningful and predictive ones.
For a deeper look at features and the elements of a predictive model, download the white paper: Third-Party Data: The Missing Ingredient to Predictive Modeling Success
Predictive Modeling Use Cases with Feature Engineering
One elementary example for a predictive modeling use case is a spam filter for email. The model would typically be trained in a binary fashion — spam or not spam. But to get there, features must be defined and engineered in training data so the model knows what to look for when classifying emails as spam or not.
Determining a business’ high-value customers is a more complicated use case, requiring the need to evaluate many features. For instance, a global food delivery company asked Mobilewalla to help it better model high-value customers.
The delivery company had some basic features taken from its records, such as order amount and order frequency for each customer. Mobilewalla then enriched the first-party data with additional features like daily commute distance, age, gender, restaurant visit frequency, supermarket visit frequency, and more.
To learn more about the determining high value customers, download our case study:
How to Increase the Value of AI with Data
Improve Your Predictive Modeling with Data Enrichment and Feature Mart with Mobilewalla
Businesses and data scientists around the world rely on Mobilewalla for trusted third-party data, data enrichment, and predictive modeling solutions. Informed by our deep data science and machine learning experience, Mobilewalla proprietary solutions help organizations get the most out of their AI investments by making the feature engineering process more systematic and procedural, decreasing the cost and time of building highly predictive features and delivering resilient, accurate machine learning models.
Mobilewalla’s Feature Mart offers more than 300 sophisticated, highly predictive features that can be used to model a variety of business outcomes. These features are predefined across nine key categories and can be applied across industries and use cases. Mobilewalla also provides the data behind the features to train and validate models, and the data science services to augment your internal teams and improve your outcomes.
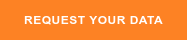