Artificial intelligence (AI) and its impact on business innovation continues to grow across industries. There’s a booming demand to add solutions that elevate consumer intelligence as customer-centric organizations realize the value of adding AI to the tools and insights that help to improve the customer experience.
From improved service personalization to advanced business insights, AI is finding its place as the next phase for organizations. However, data quality and consistency challenges have become significant barriers to AI development.
AI comprises two major components, code (models) and data. Therefore, when data scientists and analysts build predictive models, they subscribe to bifurcated schools of thought for developing AI systems: model-centric and data-centric.
From model-centric AI to data-centric AI
What is model-centric AI
The model-centric approach is the process of increasing algorithm sophistication in an attempt to improve ML model performance. It’s a common practice as data scientists can refine algorithmic code or use different modeling techniques to iterate on model improvements.
Previous and current AI applications are primarily developed with this method, as it's a common belief that model sophistication is the definitive way to increase AI effectiveness. However, model building and maintenance can be complicated and requires advanced skills and resources to manage more sophisticated models and ultimately produce improved results.
What is data-centric AI
The data-centric approach is the view that data is the key differentiator that will positively influence the performance of their ML models. A data-centric organization requires the philosophical understanding that the topic of data needs to be involved in every business decision at every employee level.
Producing AI solutions in a data-centric environment focuses on investments into data breadth, depth and quality , where the benefits are system-wide. With improved data, fewer resources and time are spent adjusting individual models to drive results but instead can have a significant impact on several models that may use the refined data.
Why data-centric AI is a more resilient practice
In our white paper How to Increase the Value of AI with Data, we helped our client improve AI efficiency by comparing the performances of two algorithms: a simple algorithm, enriched with Mobilewalla’s consumer data and a sophisticated algorithm with only first-party data. This test allowed us to discover whether the model-centric or data-centric approach is the most efficient in achieving our client’s business goals.
Mobilewalla isn’t the only party championing the data-centric AI approach. The data science community is starting to embrace this concept with the influential data scientist and Landing AI founder, Andrew Ng, leading the campaign for data-centric AI. He hosted a recent talk that also tested the two approaches in a steel defect example and proved that the data-centric approach had a more substantial success rate.
Enabling data-centric AI for consumer organizations
Consumer behavior is dynamic and is constantly changing, which reinforces that the data itself needs to be continuously enhanced to maintain model efficiency.
As data scientists and analysts agree that data drives AI success, it’s often just viewed as a one-time event1. Over time, it may become deprioritized by the iterative loop of model tweaking. When there isn’t a discerning eye on the data being used, oversight may cause potential problems in model resiliency due to data drift.
In today’s digital landscape, customers now expect predictability and convenience when interacting with brands, they expect these brands to know and understand them and to engage accordingly. When the key to a superb customer experience lies within highly predictive models, having a data-centric AI focus enables growth and adaptability for them.
Download our white paper to learn more about how a data-centric focus improves AI. >
Data-centricity requires choosing the right data provider
The consumer data ecosystem is crowded, and no two data providers offer the same quality, quantity, breadth, or depth of data. When choosing the right data provider to power ML models, organizations need to find a provider that aligns with the data-centric approach.
Mobilewalla, a leading innovative data solutions provider, has been named as a sample vendor to consider in the Gartner Hype Cycle™ for Artificial Intelligence, 20222 report for data-centric AI. We help organizations better understand their customers by enabling them to build accurate AI and ML models that analyze and predict consumer behavior to drive business results.
Connect with our data experts to learn more about Mobilewalla’s feature-rich offerings from the industry’s most comprehensive consumer behavior and demographic data repository.
GARTNER and HYPE CYCLE are registered trademarks and service marks of Gartner, Inc. and/or its affiliates in the U.S. and internationally and are used herein with permission. All rights reserved. Gartner does not endorse any vendor, product or service depicted in its research publications and does not advise technology users to select only those vendors with the highest ratings or other designation. Gartner research publications consist of the opinions of Gartner’s Research & Advisory organization and should not be construed as statements of fact. Gartner disclaims all warranties, expressed or implied, with respect to this research, including any warranties of merchantability or fitness for a particular purpose.
1Patel, Harshil. “Data-Centric Approach vs Model-Centric Approach in Machine Learning” Neptune Blog, Neptune.AI, 22 Jul. 2022, https://neptune.ai/blog/data-centric-vs-model-centric-machine-learning
2Gartner, Hype Cycle for Artificial Intelligence, By Analyst(s): Afraz Jaffri, Farhan Choudhary, 2022. Data-centric AI analysis by: Svetlana Sicular.
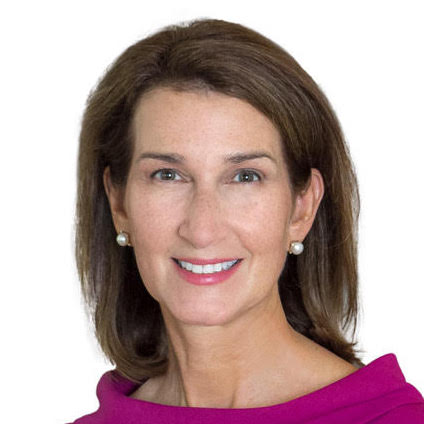